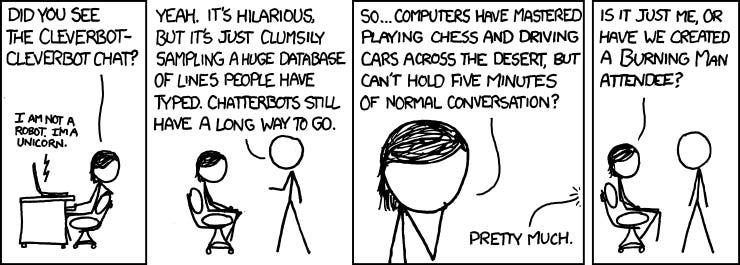
Hamilton Helmer’s 7 Powers is one of the more popular strategy frameworks, in which he highlights seven key “powers” that help companies build defensibility and become enduringly profitable businesses: Scale Economies, Network Economies, Counter-Positioning, Switching Costs, Branding, Cornered Resources, and Process Power. If you aren’t familiar, there is an excellent overview by NFX, a couple of episodes of Acquired with Helmer, and (gasp) the physical book.
No framework is perfect, but I’ve been considering the current AI wave and opportunities for startups through this lens and wanted to summarize my thoughts. As with any time a VC applies a framework, these are general hypotheses and not explicit recommendations on company strategy from someone claiming to be in the arena. (I think we can now lay that joke to rest with the alien corpses from Peru.)
Scale Economies
When companies make high fixed-cost investments, their per-unit costs decline substantially as their customer base increases, making it difficult for smaller competitors to challenge them. One of the canonical examples Helmer offers here is Netflix, which invested heavily in exclusive content early on.
In AI, the obvious comparisons are GPU production (Nvidia), foundation model providers, and GPU clouds like those built by CoreWeave and Lambda. However, the risk with some of the GPU clouds is that they are buoyed by temporary supply constraints, neglect to invest in fundamental innovations, and therefore don’t achieve materially greater scale than their competitors, ending up more like OEMs with minimal profit margins longer-term.
I’m particularly interested in how data acquisition can drive economies of scale. Companies that harness this power can spread those upfront costs around large user bases, similar to Netflix’s content strategy, and leverage LLMs to create richer product experiences on top of their unique data sets. Within vertical applications, Apollo, AutogenAI, Clay, and ZoomInfo are a few that come to mind, while Perplexity is taking a more horizontal approach to organizing the world’s knowledge.
Network Economies
The traditional definition of a network effect is when the value of the network increases with each additional user. As Helmer points out, this tends to lead to “winner take all” situations more so than the other powers.
It’s already near-impossible to displace an entrenched network, and I think this will become more difficult going forward. Established networks can leverage LLMs to improve their product experience overnight through improved recommendations, new interaction methods, or by supporting creative experiences directly on their platforms. Only last week, Roblox rolled out a toolbox for generative AI creators – an announcement likely to be the first of many, and I’m excited to see what Reddit and Discord roll out in the coming months.
If established networks stand to benefit more from AI than startups, how can startups compete within this new world order?
I’m not going to give the standard VC advice to “build a platform!” because (1) what company doesn’t want to be a platform?, (2) it downplays how incredibly difficult building a platform can be, and (3) most successful platforms started as amazing stand-alone products before expanding. OpenAI’s plugins will be more successful than others because of the size of their developer base, not because they are inherently better products.
However, I do believe that startups have a fighting chance against established networks if they invest in building a marketplace component early on. These can be transaction-based, like Replit’s bounties; or they can supercharge content creation by the community, like Polycam, Luma, and Replicate. Either way, this path increases the vectors through which users derive value from a product, efficiently seeds the network, and buys time before you need to compete with incumbents head-on.1
Counter-Positioning
According to Helmer, counter-positioning involves a smaller company innovating by using a business model that would be unprofitable for a larger business and/or cannibalize that business’s existing revenue stream.
One area I’m curious about is next-generation hardware. Recently, many startups have focused on building an integrated HW/SW solution, where a software subscription offsets the lower gross margins from commodity hardware. Could AI reverse this trend? Frameworks like ggml enable local inference and could push intelligence back to devices and away from centralized systems, resulting in higher-margin device sales with cheaper software services on top.
More generally, with the shift to consumption-based pricing, we saw increased alignment between the value generated, value captured, and cost-to-serve. As we move from Software 2.0 to 3.0, I believe we’ll see a new pricing model emerge that strikes a similar balance and allows AI-native companies to counter-position against established companies. Token-based and output-based pricing models both have potential given their cost-value alignment, similarity to consumption-based pricing, and dependency on purpose-built architectures, but it’s unlikely we’ll reach critical adoption of a new business model in the next year or two.
Switching Costs
I believe switching costs have significantly reduced as every company becomes a software company. Long, manual implementations have been replaced by “XaC” (X as Code), which results in more scalable, source-control-based solutions and makes migrating to new products more accessible. Today, we see that playing out with foundation models, where many engineering leaders prioritize interoperability and self–managing early on, especially at companies with data exfiltration concerns.
Products that require implementations from IT/technical teams, but that are accessed by business users on a weekly basis, have been more immune to this. I’m excited by the potential for startups to finally displace companies like SAP that have historically benefitted from high switching costs due to lengthy implementation cycles. The next ERP or CRM product could provide a fleet of agents to automate the initial configuration and most of the migration to a legacy provider; it’s also possible that a new, foundation model-based semantic layer could synthesize data from these legacy products and render them far less valuable.2
Branding
We all know companies that have succeeded due to solid and long-lasting brands. Typically, investors value this power because those companies can consistently charge more and tend to be a buyer’s first and safest choice.
OpenAI is the obvious brand of record within AI. Other foundation model providers are demonstrating remarkable rates of improvement but are falling into the trap of positioning themselves as “similar performance to OpenAI but cheaper” or “OpenAI but self-managed,” which could soon be rendered obsolete with the next iteration of GPT. And what happens to all the LLM tooling if OpenAI builds cheaper models, better fine-tuning, or better support for RAG?
If, like me, you believe that switching costs are generally decreasing, then companies will need to place an even greater emphasis on branding in the years to come. In infra, the default approach is to tout a high-quality developer experience, but I think this is increasingly insufficient as incumbents roll out magical experiences like Github Copilot.3
Instead, I think branding and network economies are two sides of the same coin; in both cases, your product gets propelled to new heights by the strength of its community, while competitors make the mistake of framing their products relative to your brand rather than on their own merits. Users are always your best salespeople.
Cornered Resource
This power frequently refers to teams with unique talent density and/or brain trusts at the top of an organization that consistently produce innovative products. Pixar is the canonical example here.
In AI, DeepMind/Google Brain have cornered vital talent for the last decade, but it appears that OpenAI has reached that same tier. However, given the amount of venture funding available for ex-OpenAI researchers in the past two years alone, I imagine this will become a “competitive resource” rather than a “cornered resource” in the coming years.
Process Power
From the outside, this power seems nebulous. In overly simplified terms, Process Power is the operational excellence that supercharges a team and results in consistently superior product quality. Toyota’s production process is an excellent example. On the surface, it’s not rocket science, and any competitor could adopt a similar approach. However, it requires complete buy-in and historical knowledge to run correctly.
Tactical demonstrations of process power from startup land include shipping with unmatched velocity, creating robust internal pipelines from the early days that increase developer efficiency, or demonstrating true customer obsession.
Process power is generally rare, but I think Midjourney and Eleven Labs demonstrate it. Whether it’s due to richer data sets, improved post-processing, better fine-tuning, or more likely a combination of them all, both companies have some secret sauce that consistently results in more “wow” reactions than anyone else.4 Even if someone wrote an HBR article about them, I doubt anyone could replicate their success.
I know it’s easy to make fun of frameworks, but I think 7 Powers is constructive for evaluating larger trends like what we’re seeing in the venture ecosystem today. Some of the powers could help evaluate startups and sectors in real-time (scale economies, network economies, counter-positioning, branding), while others are more likely to emerge retrospectively (switching costs, cornered resources, and process power).
If I could offer a few additional reflections:
Solving deep technical challenges up-front, and then compounding on those investments, is the best determinant of a company’s ability to generate scale economies and process power
A product is only as strong as its community
Counter-positioning isn’t just a pricing change; it relies on a purpose-built architecture to uniquely enable a different business model
I think we all may be underestimating OpenAI and Midjourney
Real magic happens when a user can get value from the product by doing solo work, collaborating effectively while still inside the product, and even interacting with people outside the organization. Creativity and productivity tools will do this through templates or UGC, Snowflake has done this through data sharing, etc.
By extension, we could also see this play out with legacy BI tools
There are always exceptions to this, such as Modal, but the bar is getting really high for what constitutes a great developer experience
Perhaps we need to create a new metric called the Owen Wilson Index that tracks how many “wows” each release gets on HN, Twitter, etc.